Retail
Extending the capabilities of Healthcare with AI and Sparkflows
Sparkflows accelerates healthcare delivery by improving patient outcomes, reducing costs, and enhancing the overall quality of care through a Best-In-Class AI-powered Business-driven
Self-Service Data & AI Solution building Platform.
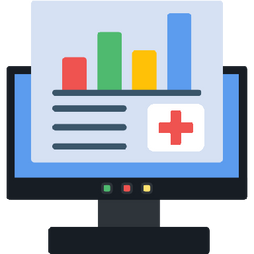
Business Challenges
Retail Business needs to address Data challenges and adopt AI-driven culture










Competition and Market Saturation
Many markets already saturated with established players
It’s challenging for a Retailer to differentiate from others and gain market share.
Customer Acquisition and Retention
Retaining customers and building brand loyalty is challenging for sustained success.
Supply Chain and Inventory Management
Efficiently managing inventory and ensuring a smooth supply chain process are critical for avoiding stockouts, overstocking, and shipping delays, which can negatively impact customer satisfaction.
Fulfillment and Shipping
Providing fast and cost-effective shipping options while maintaining product quality and minimizing shipping costs is a continuous challenge for e-commerce businesses.
Personalization and Customer Experience
Consumers have high expectations on personalized shopping experiences. e-commerce businesses need to be really well-equipped with data analytics and AI to deliver relevant product recommendations and tailored experiences.
Returns and Refunds
Managing product returns and processing refunds efficiently can be complex and impact the overall profitability of an e-commerce business.
Digital Marketing and Advertising
Effectively reaching the target audience and standing out in a crowded digital landscape require strategic digital marketing and advertising efforts.
E-commerce businesses need to adapt quickly to changing market dynamics and consumer preferences to stay competitive and successful.
Sparkflows Solution

Healthcare Data Products
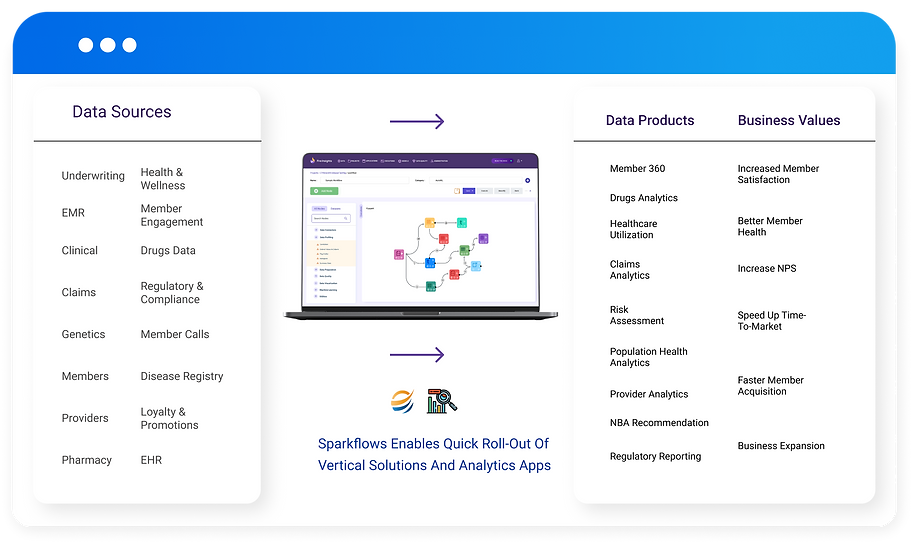
Build solutions using pre-built templates
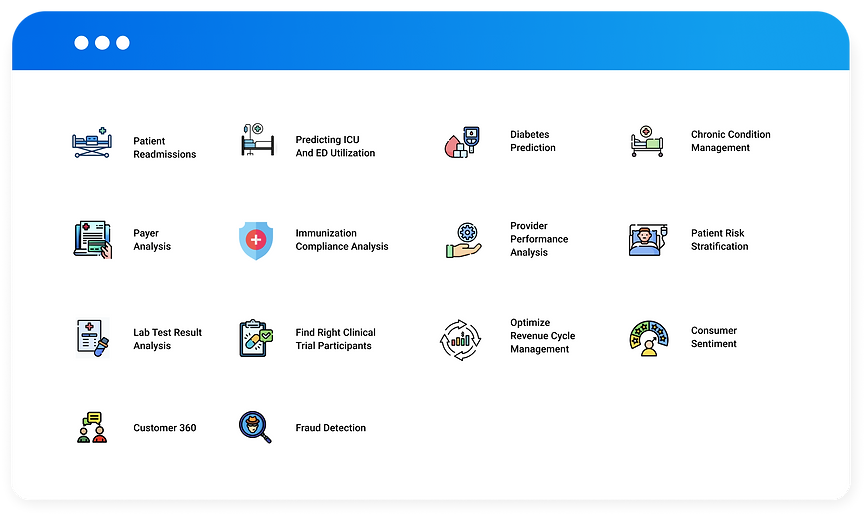
CASE STUDY
Sparkflows at a large Healthcare Company
Company Objective

A very large healthcare insurance company operating in all states of US had a requirement to speed up business case development and boost overall productivity and engineering efficiencies.
The data engineers and analysts in each region need to be empowered with self-serve advanced analytics and deliver top quality results quickly .
Business Use Cases

Customer had identified several data engineering and bigdata analytics science use cases including
-
EHR data analysis
-
Patient Admission record analysis
-
Claims data analysis
Challenges

Complex & Time-Consuming Engineering process

The current data engineering processes are somewhat complex and time consuming. They involve a lots of local coding and little automation. Oftentimes lacks regulated process. Cloud automation and Compute Push-down are not easy to implement.
Business Use Cases
Customer had identified several data engineering and Big Data analytics science use cases including
EHR data analysis
Patient Admission record analysis
Claims data analysis

Getting Value Out of Data
Not enough Data Analytics and ML applications are currently in production. Hence, they need to be quickly built.

Hard to Collaborate
In the absence of a powerful Self-Service Co-development Platform, it is impossible to the users to collaborate.
Inability to scale out use cases
Need to scale from local machine to cloud. Need to boost productivity and reduce time-to-market while accelerating the solutions.
Solution
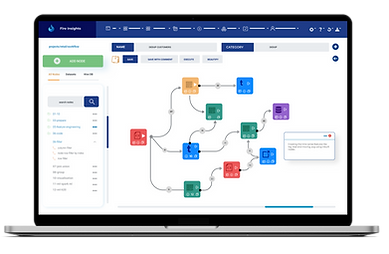

Fast development and deployment on top of AWS Datalake and EMR. Quick delivery of Business use cases and fast Time-to-Market
Sparkflows was installed in the secure air-gapped cloud environment.
Admin quickly configured the secure connections with AWS and RDS
Data Engineers could quickly connect to Data Lakes and perform scalable ETL, automatically generate distributed code.
Users could create the visual point & click workflows in minutes using existing templates.
15X
Increase in User Adoption
35X
Reduction in Time to Market
20X
Increased Collaboration
15X
Higher Accuracy of Models
They could read data from S3, DynamoDB, RDS etc., transform them and perform extensive Data Quality and generate Reports at scale.
The jobs would run distributed on EMR and automatically scheduled through Airflow and hence could scale easily.
Using the 450+ functions in Sparkflows and 80+ ML algorithms, the users were quickly able to build accurate ML models.
Multiple teams quickly collaborated with each other by sharing projects and workflows in secure manner and by pushing workflows to Github
Domain knowledge could be easily persisted and reused
Business teams quickly built Analytical Apps and powerful reports in the same project.